AI model using daily step counts predicts unplanned hospitalizations during cancer therapy
Machine-learning method identifies rapid drops in patients’ activity levels to signal need for additional care
SAN ANTONIO, October 24, 2022
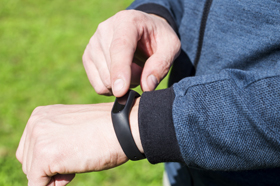
An artificial intelligence (AI) model developed by researchers can predict the likelihood that a patient may have an unplanned hospitalization during their radiation treatments for cancer. The machine-learning model uses daily step counts as a proxy to monitor patients’ health as they go through cancer therapy, offering clinicians a real-time method to provide personalized care. Findings will be presented today at the American Society for Radiation Oncology (ASTRO) Annual Meeting.
An estimated 10-20% of patients who receive outpatient radiation or chemoradiation therapy will need acute care in the form of an emergency department (ED) visit or hospital admission during their cancer treatment. These unplanned hospitalizations can be a major challenge for people undergoing cancer treatment, causing treatment interruptions and stress that may impact clinical outcomes. Early identification and intervention for patients at higher risk of complications can prevent these events.
“If you can anticipate a patient’s risk of unplanned hospitalization, you can change how you support them through their cancer treatments and reduce the likelihood that they will end up in the ED or hospital,” said Julian Hong, MD, senior author of the study and an assistant professor of radiation oncology and computational health sciences at the University of California, San Francisco (UCSF), where he also serves as Medical Director of Radiation Oncology Informatics.
Dr. Hong’s team previously demonstrated that a machine learning algorithm using health data such as cancer history and treatment plan could identify patients at higher risk of ED visits during cancer treatment, and that additional surveillance from their providers reduced acute care rates for these patients.
For the current study, he and Isabel Friesner, lead author and a clinical data scientist at UCSF, collaborated with Nitin Ohri, MD, and colleagues at Montefiore Medical Center in New York to apply machine learning approaches to data from wearable consumer devices. Dr. Ohri and his team previously collected data from 214 patients in three prospective clinical trials (NCT02649569, NCT03102229, NCT03115398). In each of these trials, participants wore fitness trackers that monitored their activity over several weeks while they received chemoradiation therapy. Trial participants had different types of primary cancers, most commonly head and neck (30%) or lung (29%) cancer.
Step counts and other data from these patients’ records were used to develop and test an elastic net-regularized logistic regression model, a type of machine-learning model that can analyze a large amount of complex information. The goal of their model was to predict the likelihood that a patient would be hospitalized in the next week, based on their previous two weeks of data.
Researchers first created the model by examining how well different variables predicted hospitalization, using data from 70% of the trial participants (151 people). Potential predictors in the model included patient characteristics (e.g., age, ECOG performance status), as well as activity data measured before and during treatment. In addition to daily step totals, the researchers computed other metrics, such as relative changes to a person’s week-by-week averages or the difference in the minimum and maximum number of steps each week.
The research team then validated the model using the remaining 30% of patients (63 people). The model that integrated step counts was strongly predictive of hospitalization the following week (AUC = 0.80, 95% confidence interval [CI] 0.60-0.90), and it significantly outperformed the model without step counts (AUC = 0.46, 95% CI 0.24-0.66, p<0.001).
“The step counts immediately preceding the prediction window ended up being generally more predictive than clinical variables. The dynamic nature of the step counts, the fact that they're changing every day, seems to make them a particularly good indicator of a patient's health status,” said Dr. Hong.
The top predictive variables in the model included step counts from each of the past two days, as well as the relative changes in maximum step count and step count range over the past two weeks.
The use of dynamic data differentiates this model from those based on clinical data like performance status and tumor histology. “One of the unique parts of this model is that it’s designed to be a running prediction,” explained Ms. Friesner. “You can run the algorithm on any given day and have an idea of a patient’s risk level one week out, giving you time to provide that additional support they need.”
This additional support is key to reducing hospitalizations, explained Dr. Hong, whether it’s scheduling more frequent follow-ups, changing something about the patient’s treatment plan or another personalized approach. “The core of what works is that this is an extra touchpoint for a doctor to see a patient. It gives the patient reassurance to know that we are watching out for them.”
“As more people begin to use wearables, the question of whether the data they are collecting could be useful arises. Our study shows there is value in having our patients collect their own health data during their everyday lives, and that we can use this data to then monitor and predict their health status,” added Ms. Friesner.
Next steps for the investigators include a more rigorous validation of the algorithm on the NRGF-001 trial (NCT04878952) led by Dr. Ohri, which will randomize patients undergoing CRT for lung cancer to treatment with or without daily step count monitoring. Physicians of patients on the step count arm will receive output from the model throughout the treatment process.
The researchers are also planning other studies to examine additional metrics collected by wearable devices, such as heart rate, and their utility in the clinic.
“Wearable devices and patient-generated health data are still relatively new phenomena, and we're still learning how they can be useful. What other information can we obtain from the many sensors in our lives? How can these metrics complement each other and work with other types of data, like electronic health record data? Different datapoints might work for better for different patients,” said Ms. Friesner.
Following the widespread adoption of telemedicine and remote care over the past several years, the need for remote monitoring via patient devices may also increase. Clinics and policymakers should keep access to these devices in mind as they grow in popularity, said Dr. Hong.
“One of the challenges when working with real-world wearable data are the economic and racial disparities that impact who owns devices that can capture this type of data. I think it’s important to develop tools that are useful for the clinic but also that are accessible to a wider range of patients.”
###
Attribution to the American Society for Radiation Oncology (ASTRO) Annual Meeting is requested in all coverage. View the meeting press kit at www.astro.org/annualmeetingpress.
See this study presented:
- Machine learning-based prediction of hospitalization using daily step counts for patients undergoing chemoradiation (Abstract 132)
- News Briefing: Tuesday, October 25, 9:00 a.m. Central time. Details here.
- Scientific Presentation: Monday, October 24, 8:40 a.m. Central time, Henry B. Gonzalez Convention Center. Details here; email [email protected] for access.
ABOUT ASTRO
The American Society for Radiation Oncology (ASTRO) is the largest radiation oncology society in the world, with nearly 10,000 members who are physicians, nurses, biologists, physicists, radiation therapists, dosimetrists and other health care professionals who specialize in treating patients with radiation therapies. For information on radiation therapy, visit RTAnswers.org. To learn more about ASTRO, visit our website and media center and follow us on social media.